60th Annual Allerton Conference on Communication, Control, and Computing
September 25 – September 27, 2024
Hosted by the Coordinated Science Laboratory at the University of Illinois at Urbana-Champaign. Each year, the Allerton Conference draws some of the brightest minds from industry, academia, and government to discuss innovation in the fields of communication, control, and computing.
This year’s conference will be held at National Center for Supercomputing Applications (NCSA)
in the engineering campus of the University of Illinois Urbana-Champaign.
Subhonmesh Bose and Rasoul Etesami
Allerton Conference Co-Chairs
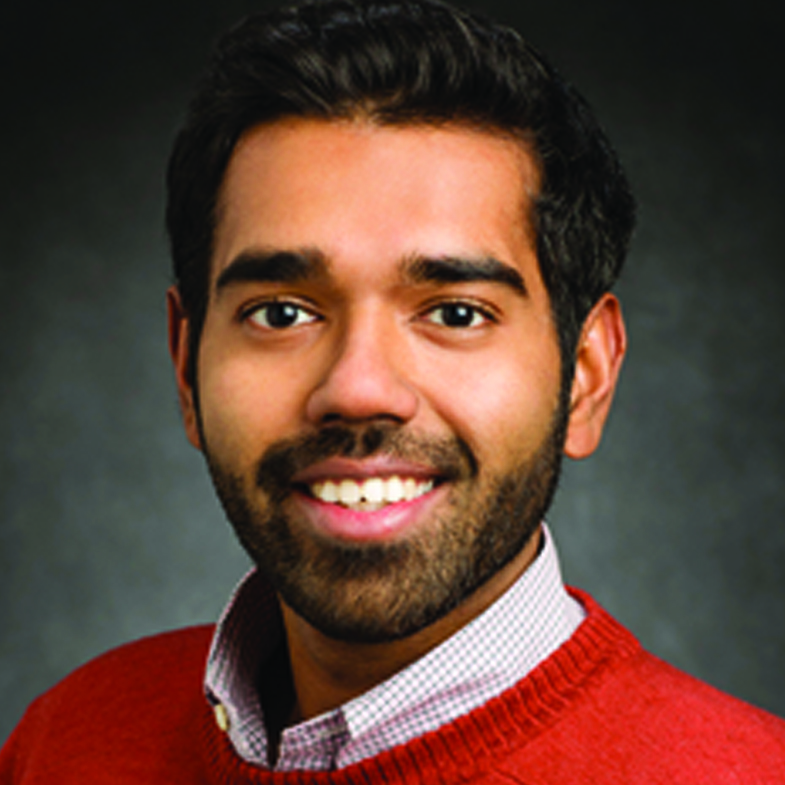
Subhonmesh Bose
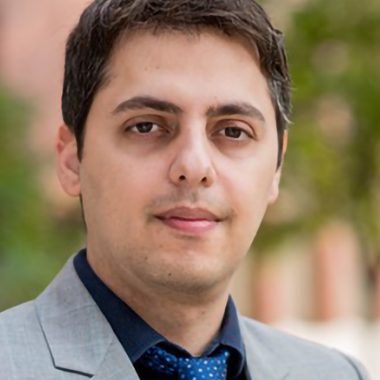
Rasoul Etesami
Plenary Speaker
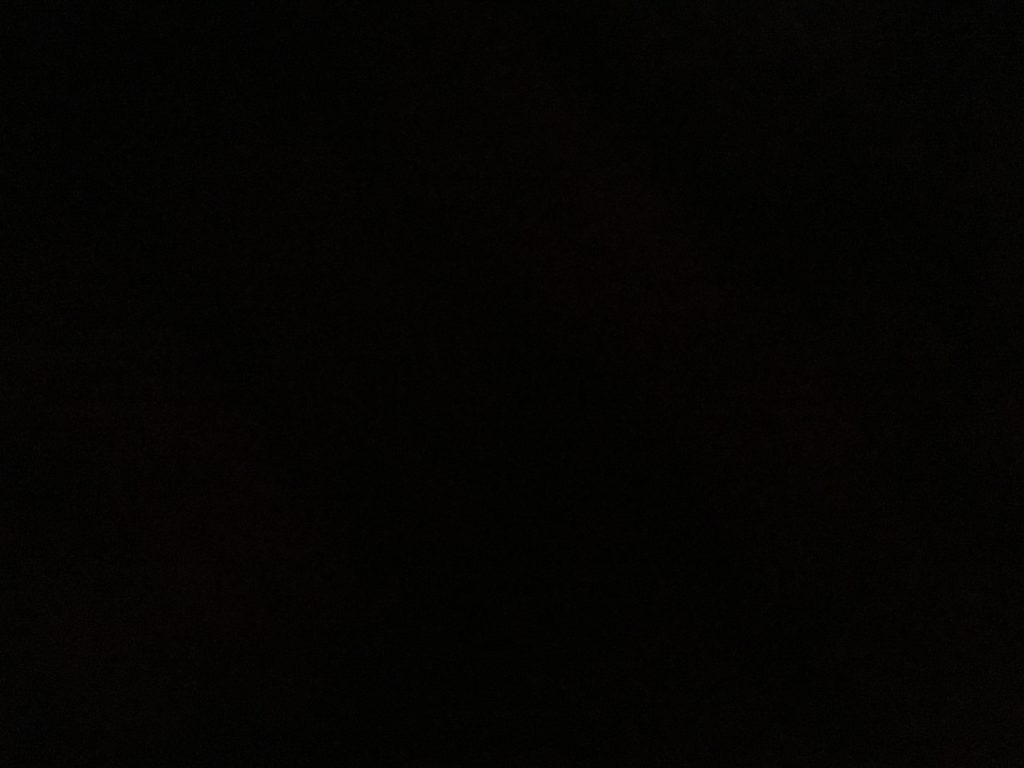
TBD
History
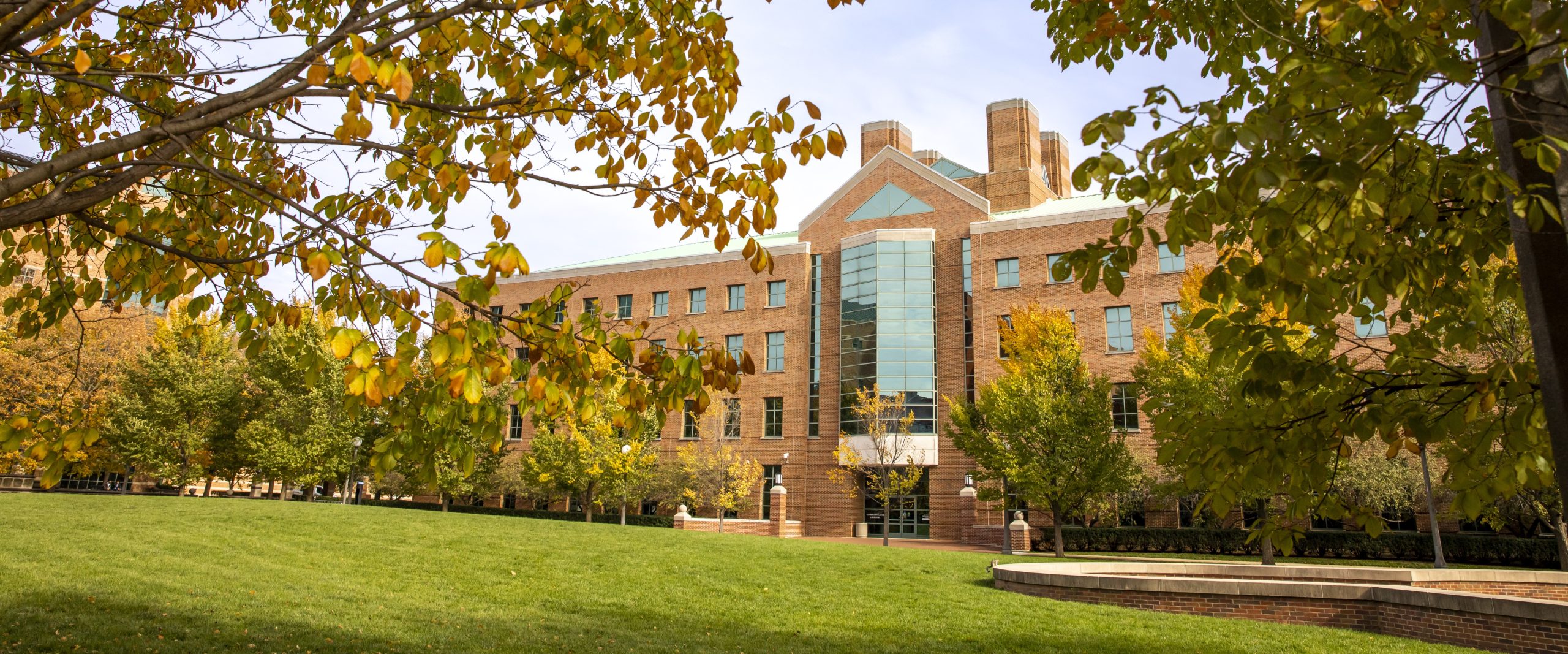
Mac Van Valkenburg, considered one of the greatest engineering educators of the past century, founded the Allerton Conference on Communication, Control, and Computing back in 1963 – then known as the Allerton Conference on Circuits and Systems.
It is one of the longest-running conferences in the systems area – and one of the most prestigious. Over the years, Allerton has drawn such illustrious academics as:
- Hungarian electrical engineer Rudolph Kalman, who co-invented the Kalman filter, a mathematical technique widely used in control systems and avionics to extract a signal from a series of incomplete and noisy measurements;
- Russian mathematician Lev Pontryagin developed a theory of characteristic classes, now known as Pontryagin classes, designed to vanish on a manifold that is a boundary;
- American engineer Jim Mulligan designed and implemented courses in VLSI at the University of California, Irvine, and whose legacy is commemorated through the IEEE James H. Mulligan, Jr. Education Medal.
Allerton Conference Today
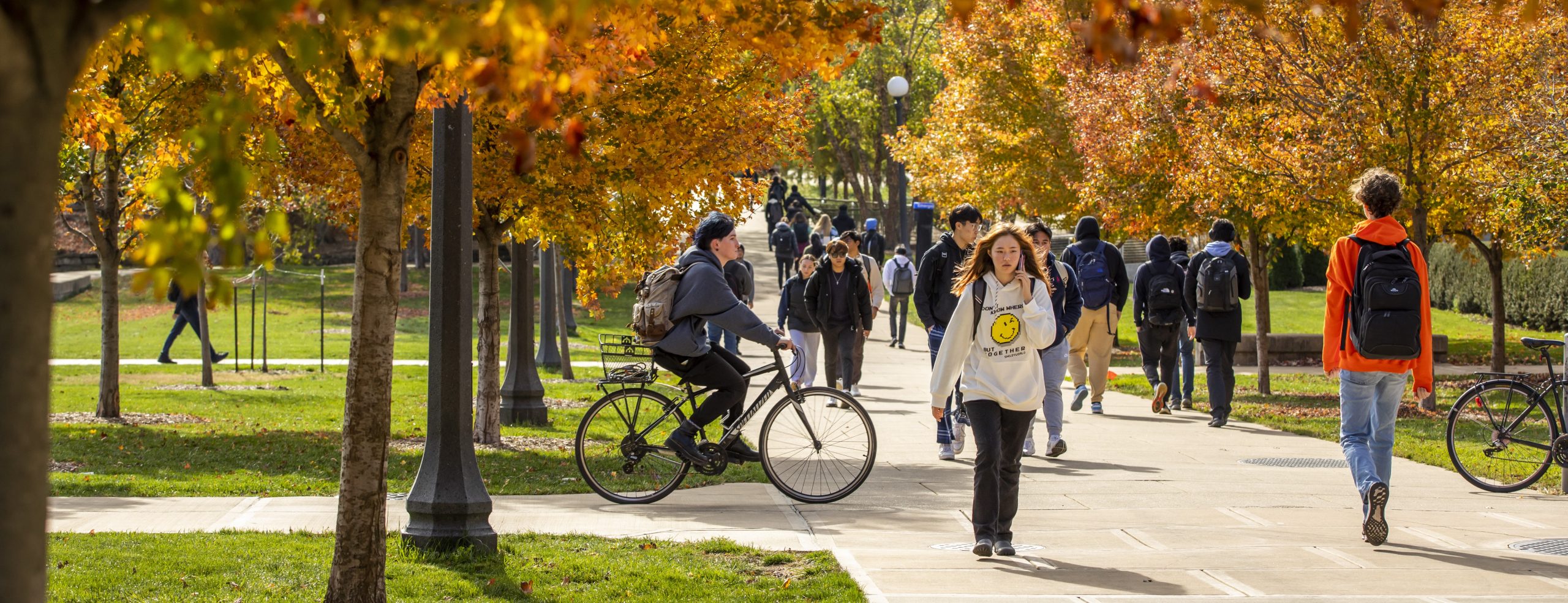
More than 50 years after its inception, the Allerton Conference continues to bring together some of the most brilliant minds in the field. For four days, participants discuss such wide-ranging topics as:
adaptive control and automation biological information systems coding and retrieval coding techniques and applications coding theory complex networked systems data analytics data storage decentralized control systems detection and estimation distributed and large-scale systems dynamic games information theory intrusion/anomaly detection and diagnosis learning and inference machine learning and learning theory multiuser detection and estimation | network coding network games and algorithms network information theory optimization performance analysis power system control and optimization pricing and congestion control reliability robotics robust and nonlinear control security and trust sensor networks sensor networks in communications signal acquisition signal models and representations statistical signal processing wireless communication systems |
The Allerton Conference has been growing each year and celebrated its 50th anniversary in 2012. During the 35th annual conference in 1997, organizers buried a time capsule with instructions to open it in another 35 years. When the capsule is opened at the 2032 conference, many aspects of communication, control, and computing will be dramatically different — but one thing will likely remain the same: The Allerton Conference will still be a gathering place for the brightest minds in the fields of communication, control, and computing.